Countries

Machine room in Kvilldal, Norway's largest hydropower plant and part of the Ulla-Førre development.
Machine learning: Teaching power plants to speak
The hydropower plants in Norway are getting older. This means costly maintenance and large reinvestments. What if the power plants could tell us what they needed and when?
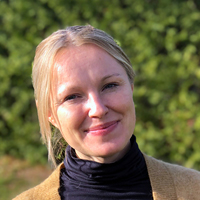
Camilla Feurst is a senior specialist in risk management in Statkraft's business area Nordics.
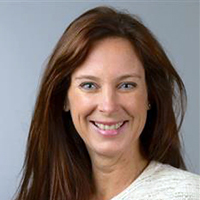
Christine Schei Liland is titled Business IT Developer and works with data modelling and project management in Statkraft's business area for CFO&IT.
Many Norwegian hydropower plants were built between 50 and 100 years ago. They have reached an age when they need more frequent maintenance and replacement of components. These are costly operations. Every year, Statkraft spends up to NOK 2 billion to maintain and raise the technical standard of valuable hydropower plants.
"With more timely and detailed information about the technical state of the hydropower plants, we can operate with shorter timeframes in maintenance planning while ensuring that we avoid mistakes," says Camilla Feurst, senior specialist risk management in Statkraft.
Crystal ball
This is where digitalisation comes into play.
"All our hydropower plants are automated, but risk assessments are still largely based on physical inspections and manual calculations. This means there is a major potential for digitalisation," she says.
In 2017, Statkraft launched a pilot project at Kvilldal hydropower plant to investigate whether advanced data analyses, algorithms and machine learning could provide new insights into the state of the power plants and be useful tools for maintenance teams.
"We were looking for a crystal ball and wanted to find out whether computer-driven models could give us the answers we needed. Our goal is always to utilise the entire life expectancy of parts and components, while avoiding failures and unplanned downtime at the power plants. With more precise risk assessments, we can also minimise planned downtime in connection with maintenance and improvements," says Feurst.
Recently, the insights gained in 2017 have been tested in new ways at Jostedal power plant.
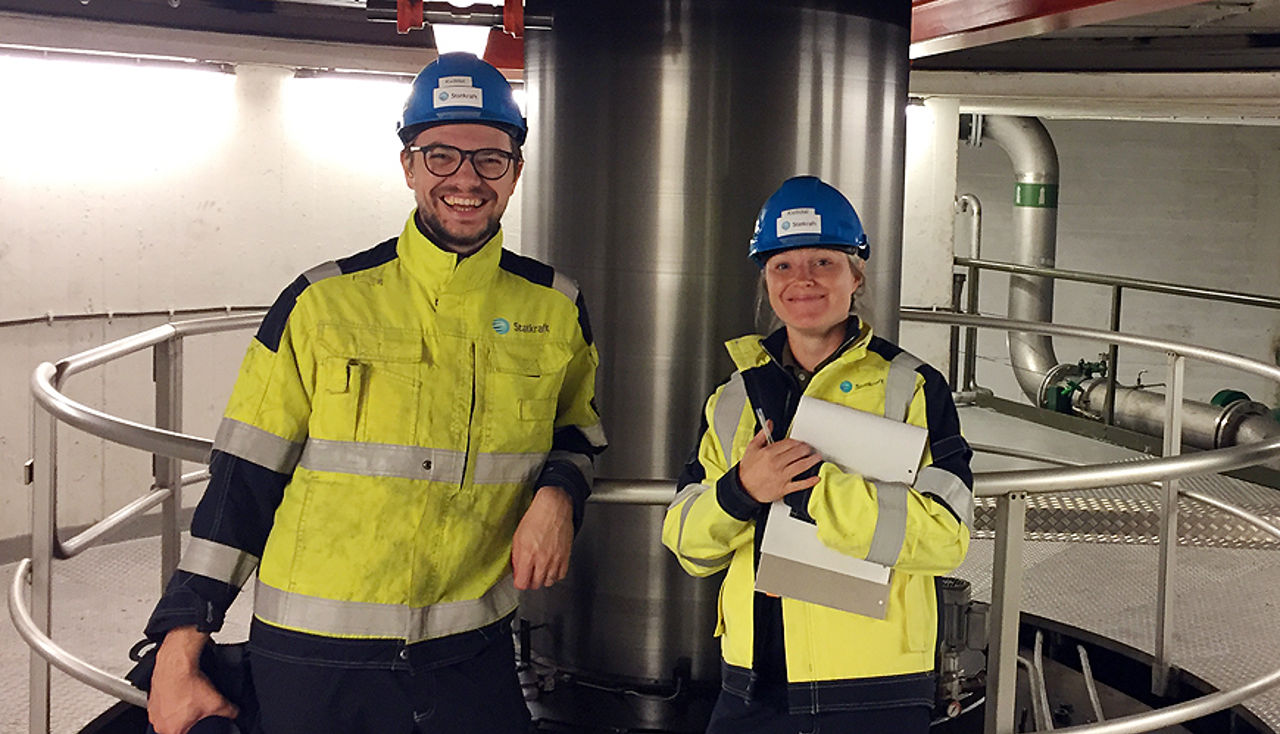
Detected changes before failure
At Jostedal, Feurst and her team has run a new machine learning “health model” to detect changes in healthy plants. Here, sensors were used to detect anomalies in what was otherwise a healthy power plant. At Kvilldal, in comparison, machine learning was used after an unexpected event had occurred.
Upon the pilot, the team at Jostedal detected minor changes in a few sensors, which took the project in a new direction.
“Although the plant was running at full speed that spring, we had identified small changes in a few sensors. We were not sure if these changes were critical or not, so we decided to stop for inspection,” says Feurst.
The inspection was set to take place three weeks later, but 12 hours before the inspection was due, a thrust bearing failed.
“Though the inspection was a bit late in this case, this was a solid validation that small changes in the data can mean something. Now we are mounting extra sensors and maintaining weekly contact with the team on-site to prevent a second failure,” Feurst explains.

Invaluable expertise
The knowledge and intuition found in the people who work at power plants is impossible to replace. In Feurst’s view, it is the combination of people’s expertise, interdisciplinary teams and the power of data that have enabled the learnings of this Statkraft team.
"The maintenance manager can place the palm of his hand on the turbine and immediately feel if the vibration is correct. But what happens when he's no longer there? It is crucial for us to have close dialogue with the maintenance team and to absorb their knowledge. People with high levels of expertise have made professional assessments in the power plants for almost a hundred years. The experience gained from this is very valuable in general, and particularly when we're going to create accurate computer-driven models," she says.
When new technology meets decades of knowledge, scepticism does occur. This has been no surprise to Feurst.
"Healthy scepticism is useful and makes the project better, but the fear that algorithms and sensors will eventually replace humans can make us less adaptable. We're still going to need people, but perhaps in different roles," she says and emphasises: "We must never forget that the models describe a reality – and that will always consist of nature and mechanics."
When power plants speak
As the Jostedal team’s experience proves, it is not too long until power plants will be able to say when they "feel" that something is not the way it should be. Sensors, data and algorithms can detect where the problem is and indicate when and how best to solve it.
“Our people on-site are constantly working to debug and decode physical components in our operations. Now we can enhance their ability to detect even the smallest changes using machine learning so that we keep our plants healthy and in full operation,” says Feurst.
"We were looking for a crystal ball and wanted to find out whether computer-driven models could give us the answers we needed. The goal is to utilise the entire life expectancy of parts and components, while avoiding failures and unplanned downtime at the power plants."